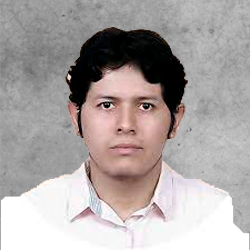
Bioengineering Department
Surgeries that use a laser as a scalpel offer several advantages such as sealing blood vessels and reducing bleeding, swelling, scarring, pain, infections, and the length of the recovery period. Before 2015, only a laser was used as a scalpel in surgical interventions in areas such as ophthalmology, dermatology and always with the laser source placed outside the human body. Recently, however, systems are being developed that incorporate a miniature robotic laser scalpel into the human body (Fig. 1). These medical devices are primarily intended to be less and less invasive to the patient. This is possible thanks to the creation of teleoperated surgical systems based on microrobots as final effectors, as an example we have an adjustable laryngoscope to be able to insert the microrobot and cameras inside the patient’s body.
Fig. 1. Concept of µRALP project. The overall strategy is to develop a common basis for the analysis, design and control of medical microrobotic systems for laser microsurgeries based on the needs and specifications coming from the medical area. [1]
To create the small-scale complex 3D structure of a microrobot (Fig. 2), flexible and rigid materials are used that combine into a monolithic flat structure that then folds to obtain a complex and rigid mechanical structure. The Smart Composite Microstructure (SCM) allows the development of microrobots because it can combine piezoelectric materials, carbon fibers and polymeric materials (polyamide) in small sizes. The advantages of SCM microfabrication technology are its simplicity in manufacturing small 3D mechanisms and its ability to create bending hinges with a greater range of motion than those made of silicon. In addition, the SCM technique produces notable improvements without causing fractures or rapid fatigue in the operating structure. Due to the manufacturing process of SCM and the nature of the materials used, uncertainties are observed in the angles, the directions of the axes and the positions of the points in the bending joints. These uncertainties greatly influence the accuracy of the microrobot’s end effector.
Very important, a small uncertainty in a joint is amplified and transmitted by the rest of the structure until the final effector (propagation of uncertainties). Unfortunately, not all uncertainties can be cancelled and a method is needed to estimate the uncertainty of the residual final effector. This significantly impacts the development of microrobots. Once the mechanical structure of the microrobot has been designed, it needs to pass multiple tests that validate its correct functioning. So microrobots that are in development must undergo several tests to obtain the required certifications for marketing and use in patients.
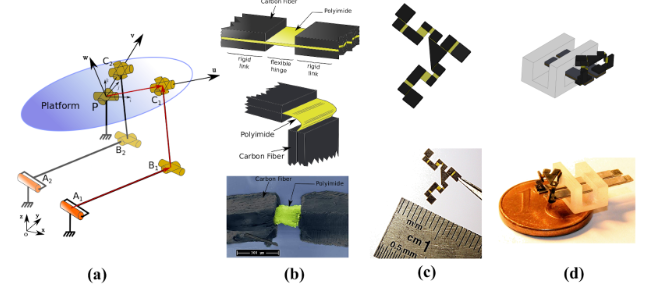
Fig. 2. Manufacture of the microrobot. (a) Operating mechanism. (b) CAD model of an extended and folded hinge with its pseudo-colored SEM image manufactured by SCM showing the carbon fiber along with the polyamide. (c) 1D design in CAD and its implementation in planar form. (D) 3D design of the folded structure and the final implementation of the microrobot. [2]
In fact, one of the main tests to validate is the precise determination of its reliability and remaining shelf life to ensure optimal performance during the surgical procedure. The Health Forecasting and Management (PHM) approach incorporates many assessment skills from observation to the decision phase. PHM systems are some of the main protagonists of the Industry 4.0 revolution whose main challenges are to efficiently detect if a component of a system has deviated from its normal operating condition or predict when some will occur failure. Efficient PHM methods promise to decrease the probability of extreme failure events, improving the level of safety of equipment such as biomedical robots operating in very crucial processes where a small deviation could be fatal to a patient’s life.
To estimate the RUL of the systems there are several methodologies and forecasting techniques and they are classified into four groups. (1) Experience-based approaches use the distribution of event records of a population of an identical element, can be implemented when historical repair and failure data are available and do not consider the indication of failure to predict the lifespan. (2) Model-based approaches use dynamic mathematical models, which can be physics-based models and statistical models. (3) Knowledge-based approaches are usually solved by human specialists, for example, expert systems and fuzzy logic systems are used for fault diagnosis. (4) Data-based approaches are based on statistical and machine learning techniques, such as multivariate statistical methods, neural networks, Bayesian networks, and hidden Markov models.
The latter are being widely used however there are several challenges of preventive maintenance on production lines from a machine learning perspective. First, it is really difficult to acquire machine malfunction data and label the failure case in practice in the data set. Secondly, there is a lot of data to process (big data) generated by the actuators, and the processing of this big data requires a special infrastructure, expert knowledge and customized intelligent software. Finally, many technology companies do not share this type of data publicly due to data privacy and, as such, researchers cannot validate new models with more data sets.
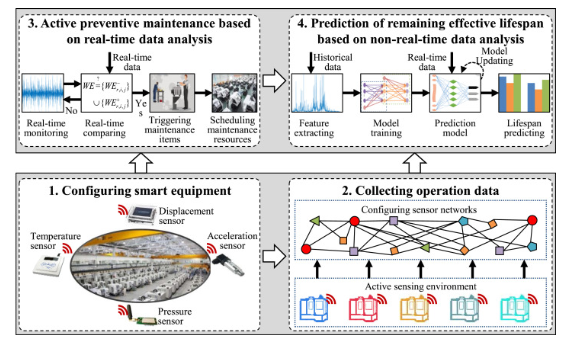
Fig. 3: Conceptual architecture for active preventive maintenance of complex equipment. It includes four main modules: (1) intelligent equipment configuration, (2) operational data collection, (3 ) active preventive maintenance based on real-time data analysis, and (4) RUL prediction based on non-real-time data analysis. [7]
Currently professors of Bioengineering of UTEC are collaborating in the development and construction of microrobots with the FEMTO-ST Institute and AMAROB Technologies, both institutions in France. If you are interested in being part of building microrobots and similar projects you expect: Join UTEC Bioengineering!
References:
- Pasaguayo, L., Z. A. Masry, and S. Lescano (2021). Degradation modeling analysis for micro-robots flexure hinges using intracorporeal surgeries. Proceedings of the 31th European Safety and Reliability Conference.
- Lescano S., Rakotondrabe M., Andreff N. Precision Prediction Using Interval Exponential Mapping of a Parallel Kinematic Smart Composite Microstructure. RSJ International Conference on Intelligent Robots and Systems, Sep 2015, Hamburg, Germany. Hal-02868200
- R. Zemouri, Z. Al Masry, I. Remadna, L.S. Terrissa, N. ZerhouniHybrid Architecture of Deep Convolutional Variational Auto-encoder for Remaining useful Life Prediction, 2020
- E.R Leighton, P.A. Cárdenas Lizana. Control System based on Reinforcement Learning applied to a Klatt-Engell Reactor. 2020 International Conference on Mechatronics, Electronics and Automotive Engineering (ICMEAE)
- Ning Wang et al. An active preventive maintenance approach of complex equipment based on a novel product-service system operation mode, Journal of Cleaner Production, 2020.
- Kang, Ziqiu et al. “Remaining Useful Life (RUL) Prediction of Equipment in Production Lines Using Artificial Neural Networks.” Sensors (Basel, Switzerland) vol. 21,3 932. 30 Jan. 2021.
- Luca Biggio et al. Prognostics and Health Management of Industrial Assets: Current Progress and Road Ahead. REVIEW article Front. Artif. Intell., 09 November 2020
- Atamuradov, V., and N. Zerhouni (2017, December). Prognostics and health management for maintenance practitioners-review, implementation and tools evaluation. Journal of Prognostics and Health Management.