Author
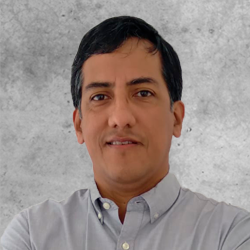
Professor in the Department of Bioengineering
What is the importance of protein folding? The structure of a protein is linked to its function and allows determining its role and therefore, the patterns that govern life. Resolving the structure of a protein can take several years and be highly expensive. Being this information crucial for the development of therapeutic drugs and biological research, protein folding is one of the bottlenecks in scientific research. Recently, a finding has shown that using artificial intelligence can accelerate the development of new drugs and understand some diseases by deciphering the spatial structure of proteins, just with the linear sequence of their amino acids. Knowing the structure of proteins allows us to understand how the processes in the cell work. Diseases like Alzheimer and Parkinson or prions are related to the misfolding of proteins. If the structure of a protein is known, this knowledge can be applied to the implementation of therapies or development of enzymes for functions of interest. However, it is necessary to solve the enigma of folding, for this there are currently expensive techniques such as nuclear magnetic resonance, X-ray crystallography, and cryo-electron microscopy.
The international competition CASP (critical assessment of protein structure prediction) has been held since 1994 in order to elucidate protein folding using computational methods. During its early years, only interaction methods have been proposed using similar natural molecules through algorithms such as Rossetta’s or taking advantage of the capabilities of molecular “docking”. However, the end product is just a naturally structured molecule that has a “high probability” of being biologically active. In many cases, after numerous and expensive in vitro tests, a group of candidates are obtained to be analyzed in animal models. Similar efforts involved creating a social network to “gamify” the algorithms through portable applications such as “Folding at Home” and Foldit. These are ways to use social networks and “gamify” algorithms to accelerate the production of new structural protein models using the computational power of a community.
In 2018, the London-based company DeepMind, when participating in the CASP13 competition, used the ALPHA FOLD 2 system to elucidate the structure of a protein. This English company does not belong to the field of biological scientific research, but uses “deep learning” (DL) models to solve practical problems. In this competition, DeepMind demonstrated that by applying DL algorithms, automated molecular dynamics can be obtained to infer protein folds with a medium computational cost. In 2018, for the first time, DeepMind won first place in the CASP13 protein computational folding competition. In 2020 the new version of DeepMind known as AlphaFold 2 for the second time, outperformed all teams in the CASP14 competition.
Predicting the 3D structure of a protein from its linear amino acid sequence is a complex biological problem that must involve understanding the spatial interaction dynamics of amino acids. If one decides to evaluate all possible configurations, the Levinthal Paradox is reached with a number of 10,300 possible configurations. Which would be impossible to process since it would take a time greater than the age of the universe to decipher the correct conformation.
DeepMind’s proposal is to solve the problem of folding based on the conversion of image´s pattern. It is proposed to find the distances between all amino acids to form a correlation matrix. A first image is obtained, which is a heat map with all the amino acid distances called PDM (“protein distance matrix”). An artificial intelligence algorithm can process molecular dynamics parameters using PDM and a heat map (Figure 1A). Additionally, crucial amino acids are found in the spatial structure using MSA (“multiple sequence alignment”). MSA is a massive alignment where proteins are compared with an evolutionary relationship. In alignment, it is checked whether an amino acid changes (“evolves”) due to a mutation and that another amino acid of the same protein usually changes in parallel. These parallel changes occur because they physically interact in the protein and must change together to maintain the structure of the protein (structural coevolution). Therefore, DeepMind proposed to combine the global distances of all amino acids using PDM with distograms (Figure 1B), and at the same time identify the physically interacting amino acids identified by MSA to infer the spatial structure of proteins. All these data are analyzed using convolutional neural network algorithms, used for image recognition. The model “learns” to generate an image like Figure 1B; A representing the three-dimensional structure of the protein (distogram). The distogram is an image that is a simple representation of the three-dimensional distance of all amino acids. Using a second artificial intelligence algorithm called “gradient descent”; the structure is adjusted to fold it optimizing the torsion angles and the physically interacting angles of the related amino acids in coevolution.
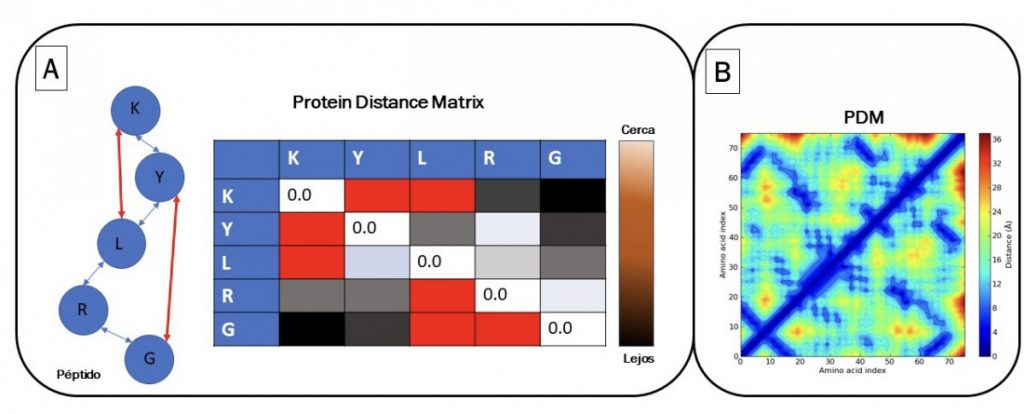
Source: Alberto Donayre
To train the model the proteins used are the ones deposited in the Protein Data Bank (PDB) this were generated by more expensive techniques such as crystallography, NMR or Electro-Cryo-Microscopy (ECM). AlphaFold recognizes folding patterns by comparing PDM, MSA data and PDB deposited structures obtaining highly accurate results. To measure this precision, the “global distance test” (GDT) index is used; which compares natural distances with those predicted on a scale from 0 to 100, and indicates the percentage of amino acid residues in the correct position [8]. Knowing that X-ray crystallography and NMR obtain GDT values of 90 or higher, in the case of AlphaFold 2 a value of 92.4 GDT was obtained using the ORF8 protein of SARS-CoV2. This result saw DeepMind win the CASP14 competition in 2020 (Figure 2). Although AlphaFold 2 still requires a moderate computational cost, the GDT values obtained are already an important technological advance and an example of how artificial intelligence can solve current biological problems. Additionally, it is also proposed to develop “inverse protein folding”; which is a strategy that allows the “optimal” linear amino acid sequence to be deduced if a theoretical protein structure that fulfills a relevant function is known.
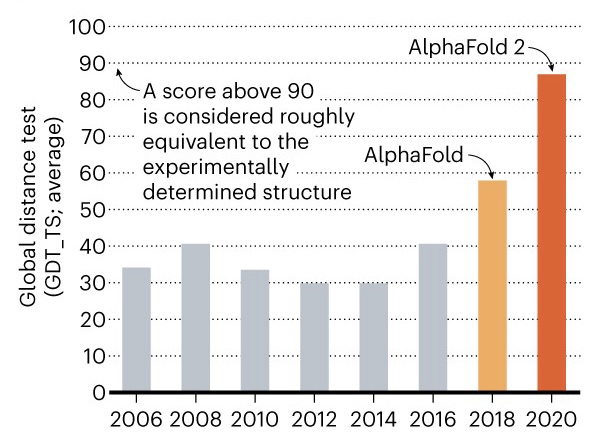
Source. Nature (2020).
To date, the limitation to the de novo protein production process is the availability of crystallized structures described in databases as Protein Data Bank (PDB). Only with this collection, structural biologists infer similar 3D molecules supported by spatial comparison algorithms and thus predict a biological function. The game has changed and AlphaFold offers a powerful alternative to predict the 3D structure of proteins and study their function; For this reason, it has been classified as the most relevant technological advance of the year 2020 (Figure 3).
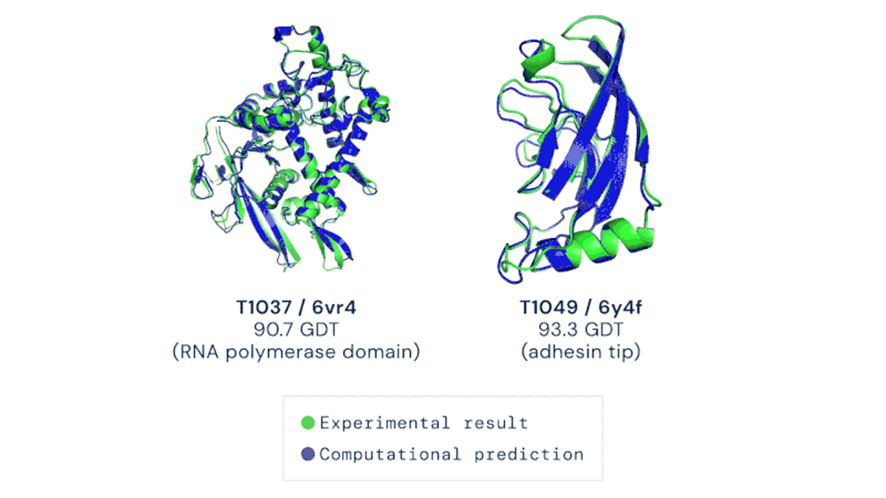
Source. Nature (2020).
Bibliographic references:
1.- Das Gupta D, Kaushik R, Jayaram B. Protein folding is a convergent problem! Biochem Biophys Res Commun. 2016 Nov 25;480(4):741-744. doi: 10.1016/j.bbrc.2016.10.119. Epub 2016 Oct 28. PMID: 27983988.
2.- Ewen Callaway. ‘It will change everything’: DeepMind’s AI makes gigantic leap in solving protein structures. Nature 588, 203-204 (2020); doi: https://doi.org/10.1038/d41586-020-03348-4.
3.- Sikder AR, Zomaya AY. An overview of protein-folding techniques: issues and perspectives. Int J Bioinform Res Appl. 2005;1(1):121-43. doi: 10.1504/IJBRA.2005.006911. PMID: 18048125.
4.- Carol A. Rohl, Charlie E.M. Strauss, Kira M.S. Misura, David Baker. Protein Structure Prediction Using Rosetta. Methods in Enzymology. Academic Press, Volume 383, 2004, Pages 66-93, ISSN 0076-6879. https://doi.org/10.1016/S0076-6879(04)83004-0.
5.- Pande lab (August 2, 2012). «Folding@home Open Source FAQ». Folding@home. foldingathome.org. Archived from the original (FAQ) on March 3, 2020. Retrieved July 8, 2013.
6.- Cooper S, Khatib F, Treuille A, Barbero J, Lee J, Beenen M, et al. (August 2010). «Predicting protein structures with a multiplayer online game». Nature. 466 (7307): 756–60.
7.- Markoff J (10 August 2010). «In a Video Game, Tackling the Complexities of Protein Folding». The New York Times. Retrieved 12 February 2013.
8.- Senior, A.W., Evans, R., Jumper, J. et al. Improved protein structure prediction using potentials from deep learning. Nature 577, 706–710 (2020). https://doi.org/10.1038/s41586-019-1923-7.
9.- Levinthal, Cyrus (1968). «Are there pathways for protein folding?». Journal de Chimie Physique et de Physico-Chimie Biologique 65: 44-45.